4/2017 - 10 |
K-Linkage: A New Agglomerative Approach for Hierarchical ClusteringYILDIRIM, P.![]() ![]() ![]() ![]() ![]() ![]() |
Extra paper information in ![]() ![]() ![]() |
Click to see author's profile in ![]() ![]() ![]() |
Download PDF ![]() |
Author keywords
clustering, data mining, data processing, knowledge discovery, unsupervised learning
References keywords
clustering(33), hierarchical(31), applications(11), systems(9), agglomerative(8), fast(7), data(7), algorithm(7), linkage(6), jeswa(6)
Blue keywords are present in both the references section and the paper title.
About this article
Date of Publication: 2017-11-30
Volume 17, Issue 4, Year 2017, On page(s): 77 - 88
ISSN: 1582-7445, e-ISSN: 1844-7600
Digital Object Identifier: 10.4316/AECE.2017.04010
Web of Science Accession Number: 000417674300010
SCOPUS ID: 85035794377
Abstract
In agglomerative hierarchical clustering, the traditional approaches of computing cluster distances are single, complete, average and centroid linkages. However, single-link and complete-link approaches cannot always reflect the true underlying relationship between clusters, because they only consider just a single pair between two clusters. This situation may promote the formation of spurious clusters. To overcome the problem, this paper proposes a novel approach, named k-Linkage, which calculates the distance by considering k observations from two clusters separately. This article also introduces two novel concepts: k-min linkage (the average of k closest pairs) and k-max linkage (the average of k farthest pairs). In the experimental studies, the improved hierarchical clustering algorithm based on k-Linkage was executed on five well-known benchmark datasets with varying k values to demonstrate its efficiency. The results show that the proposed k-Linkage method can often produce clusters with better accuracy, compared to the single, complete, average and centroid linkages. |
References | | | Cited By |
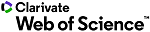
Web of Science® Times Cited: 16 [View]
View record in Web of Science® [View]
View Related Records® [View]
Updated today
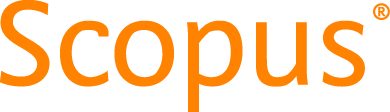
SCOPUS® Times Cited: 20
View record in SCOPUS® [Free preview]
View citations in SCOPUS® [Free preview]
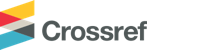
[1] DP-k-modes: A self-tuning k-modes clustering algorithm, Xie, Juanying, Wang, Mingzhao, Lu, Xiaoxiao, Liu, Xinglin, Grant, Philip W., Pattern Recognition Letters, ISSN 0167-8655, Issue , 2022.
Digital Object Identifier: 10.1016/j.patrec.2022.04.026 [CrossRef]
[2] Diabetes subtypes classification for personalized health care: A review, Omar, Nashuha, Nazirun, Nisha Nadhira, Vijayam, Bhuwaneswaran, Wahab, Asnida Abdul, Bahuri, Hana Ahmad, Artificial Intelligence Review, ISSN 0269-2821, Issue 3, Volume 56, 2023.
Digital Object Identifier: 10.1007/s10462-022-10202-8 [CrossRef]
[3] Two-level clustering of UML class diagrams based on semantics and structure, Ma, Zongmin, Yuan, Zhongchen, Yan, Li, Information and Software Technology, ISSN 0950-5849, Issue , 2021.
Digital Object Identifier: 10.1016/j.infsof.2020.106456 [CrossRef]
[4] Dual-granularity weighted ensemble clustering, Xu, Li, Ding, Shifei, Knowledge-Based Systems, ISSN 0950-7051, Issue , 2021.
Digital Object Identifier: 10.1016/j.knosys.2021.107124 [CrossRef]
[5] End-to-End Hierarchical Reinforcement Learning With Integrated Subgoal Discovery, Pateria, Shubham, Subagdja, Budhitama, Tan, Ah-Hwee, Quek, Chai, IEEE Transactions on Neural Networks and Learning Systems, ISSN 2162-237X, Issue 12, Volume 33, 2022.
Digital Object Identifier: 10.1109/TNNLS.2021.3087733 [CrossRef]
[6] A Novel Algorithm Based on Geometric Characteristics for Tree Branch Skeleton Extraction from LiDAR Point Cloud, Yang, Jie, Wen, Xiaorong, Wang, Qiulai, Ye, Jin-Sheng, Zhang, Yanli, Sun, Yuan, Forests, ISSN 1999-4907, Issue 10, Volume 13, 2022.
Digital Object Identifier: 10.3390/f13101534 [CrossRef]
[7] Determining optimal granularity level of modular product with hierarchical clustering and modularity assessment, Li, Zhong-kai, Wang, Shuai, Yin, Wen-wei, Journal of the Brazilian Society of Mechanical Sciences and Engineering, ISSN 1678-5878, Issue 8, Volume 41, 2019.
Digital Object Identifier: 10.1007/s40430-019-1848-y [CrossRef]
[8] Confidence-Based Simple Graph Convolutional Networks for Face Clustering, Sun, Dengdi, Yang, Kang, Ding, Zhuanlian, IEEE Access, ISSN 2169-3536, Issue , 2022.
Digital Object Identifier: 10.1109/ACCESS.2022.3142922 [CrossRef]
[9] Efficient and Privacy Preserving Clustering Algorithm for Spatiotemporal Data, Mehmood, Abid, Natgunanathan, Iynkaran, Xiang, Yong, International Journal of Information Technology & Decision Making, ISSN 0219-6220, Issue 02, Volume 23, 2024.
Digital Object Identifier: 10.1142/S0219622022500110 [CrossRef]
[10] Fuzzy-Rough Intrigued Harmonic Discrepancy Clustering, Yue, Guanli, Qu, Yanpeng, Yang, Longzhi, Shang, Changjing, Deng, Ansheng, Chao, Fei, Shen, Qiang, IEEE Transactions on Fuzzy Systems, ISSN 1063-6706, Issue 10, Volume 31, 2023.
Digital Object Identifier: 10.1109/TFUZZ.2023.3247912 [CrossRef]
[11] Analysis of the Evolution of the Spanish Labour Market Through Unsupervised Learning, Luna-Romera, Jose Maria, Nunez-Hernandez, Fernando, Martinez-Ballesteros, Maria, Riquelme, Jose C., Usabiaga Ibanez, Carlos, IEEE Access, ISSN 2169-3536, Issue , 2019.
Digital Object Identifier: 10.1109/ACCESS.2019.2935386 [CrossRef]
[12] Genie+OWA: Robustifying hierarchical clustering with OWA-based linkages, Cena, Anna, Gagolewski, Marek, Information Sciences, ISSN 0020-0255, Issue , 2020.
Digital Object Identifier: 10.1016/j.ins.2020.02.025 [CrossRef]
[13] A Hierarchical Attention Network for Cross-Domain Group Recommendation, Liang, Ruxia, Zhang, Qian, Wang, Jianqiang, Lu, Jie, IEEE Transactions on Neural Networks and Learning Systems, ISSN 2162-237X, Issue 3, Volume 35, 2024.
Digital Object Identifier: 10.1109/TNNLS.2022.3200480 [CrossRef]
[14] A Survey of Data Mining and Deep Learning in Bioinformatics, Lan, Kun, Wang, Dan-tong, Fong, Simon, Liu, Lian-sheng, Wong, Kelvin K. L., Dey, Nilanjan, Journal of Medical Systems, ISSN 0148-5598, Issue 8, Volume 42, 2018.
Digital Object Identifier: 10.1007/s10916-018-1003-9 [CrossRef]
[15] Automatic Hierarchical Reinforcement Learning for Reusing Service Process Fragments, Yang, Rong, Li, Bing, Liu, Zhengli, IEEE Access, ISSN 2169-3536, Issue , 2021.
Digital Object Identifier: 10.1109/ACCESS.2021.3054852 [CrossRef]
Disclaimer: All information displayed above was retrieved by using remote connections to respective databases. For the best user experience, we update all data by using background processes, and use caches in order to reduce the load on the servers we retrieve the information from. As we have no control on the availability of the database servers and sometimes the Internet connectivity may be affected, we do not guarantee the information is correct or complete. For the most accurate data, please always consult the database sites directly. Some external links require authentication or an institutional subscription.
Web of Science® is a registered trademark of Clarivate Analytics, Scopus® is a registered trademark of Elsevier B.V., other product names, company names, brand names, trademarks and logos are the property of their respective owners.
Faculty of Electrical Engineering and Computer Science
Stefan cel Mare University of Suceava, Romania
All rights reserved: Advances in Electrical and Computer Engineering is a registered trademark of the Stefan cel Mare University of Suceava. No part of this publication may be reproduced, stored in a retrieval system, photocopied, recorded or archived, without the written permission from the Editor. When authors submit their papers for publication, they agree that the copyright for their article be transferred to the Faculty of Electrical Engineering and Computer Science, Stefan cel Mare University of Suceava, Romania, if and only if the articles are accepted for publication. The copyright covers the exclusive rights to reproduce and distribute the article, including reprints and translations.
Permission for other use: The copyright owner's consent does not extend to copying for general distribution, for promotion, for creating new works, or for resale. Specific written permission must be obtained from the Editor for such copying. Direct linking to files hosted on this website is strictly prohibited.
Disclaimer: Whilst every effort is made by the publishers and editorial board to see that no inaccurate or misleading data, opinions or statements appear in this journal, they wish to make it clear that all information and opinions formulated in the articles, as well as linguistic accuracy, are the sole responsibility of the author.