1/2022 - 1 | View TOC | « Previous Article | Next Article » |
A Hybrid Deep Learning Approach for Intrusion Detection in IoT NetworksEMEC, M.![]() ![]() ![]() ![]() ![]() ![]() |
Extra paper information in ![]() ![]() ![]() |
Click to see author's profile in ![]() ![]() ![]() |
Download PDF ![]() |
Author keywords
hybrid intelligent systems, Internet of Things, intrusion detection, learning systems, prediction methods
References keywords
learning(19), detection(17), deep(15), network(14), intrusion(13), link(7), system(6), security(6), neural(6), model(6)
Blue keywords are present in both the references section and the paper title.
About this article
Date of Publication: 2022-02-28
Volume 22, Issue 1, Year 2022, On page(s): 3 - 12
ISSN: 1582-7445, e-ISSN: 1844-7600
Digital Object Identifier: 10.4316/AECE.2022.01001
Web of Science Accession Number: 000762769600002
SCOPUS ID: 85126801506
Abstract
Internet of Things (IoT) devices have flocked the whole world through the Internet. With increasing mission-critical IoT data traffic, attacks on IoT networks have also increased. Many newly crafted attacks on IoT communication require equally intelligent intrusion detection methods to form the first step of countering the attacks. Our work contributes to intrusion detection in IoT networks, by putting state-of-the-art Deep learning methods into service. A BLSTM-GRU Hybrid (BGH) model has been designed to detect eight known IoT network attacks, based on two well-accepted CIC-IDS-2018 and BoT-IoT IoT network traffic datasets. The results of our BGH model in IoT network traffic intrusion detection have been auspicious. The accuracies of prediction on the two datasets are 98.78% and 99.99%. The f1-scores are 98.64% and 99.99%, respectively. The comparison of our results with similar previous studies showed that our BGH model has the best performance ratio (time/accuracy, time/f1-score), where time is the training time of the model. The performance of our proposed model is proof that hybrid Deep Learning methods can prove to be an innovative perspective on Intrusion Detection in IoT networks. |
References | | | Cited By |
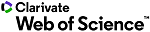
Web of Science® Times Cited: 5 [View]
View record in Web of Science® [View]
View Related Records® [View]
Updated 2 days, 8 hours ago
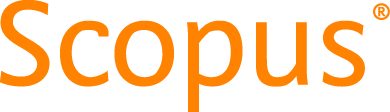
SCOPUS® Times Cited: 16
View record in SCOPUS® [Free preview]
View citations in SCOPUS® [Free preview]
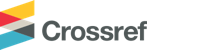
[1] Epilepsy Seizure Prediction from EEG Signal Using Machine Learning Techniques, SIDAOUI, B., SADOUNI, K., Advances in Electrical and Computer Engineering, ISSN 1582-7445, Issue 2, Volume 23, 2023.
Digital Object Identifier: 10.4316/AECE.2023.02006 [CrossRef] [Full text]
[2] Advancing IoT security: A novel intrusion detection system for evolving threats in industry 4.0 using optimized convolutional sparse Ficks law graph point trans-Net, Mathina, P.A., Valarmathi, K., Computers & Security, ISSN 0167-4048, Issue , 2025.
Digital Object Identifier: 10.1016/j.cose.2024.104169 [CrossRef]
[3] CBF-IDS: Addressing Class Imbalance Using CNN-BiLSTM with Focal Loss in Network Intrusion Detection System, Peng, Haonan, Wu, Chunming, Xiao, Yanfeng, Applied Sciences, ISSN 2076-3417, Issue 21, Volume 13, 2023.
Digital Object Identifier: 10.3390/app132111629 [CrossRef]
[4] A novel bidirectional LSTM model for network intrusion detection in SDN-IoT network, Sri vidhya, G., Nagarajan, R., Computing, ISSN 0010-485X, Issue 8, Volume 106, 2024.
Digital Object Identifier: 10.1007/s00607-024-01295-w [CrossRef]
[5] Botnetābased IoT network traffic analysis using deep learning, Singh, N. Joychandra, Hoque, Nazrul, Singh, Kh. Robindro, Bhattacharyya, Dhruba K., SECURITY AND PRIVACY, ISSN 2475-6725, Issue 2, Volume 7, 2024.
Digital Object Identifier: 10.1002/spy2.355 [CrossRef]
[6] ABCNN-IDS: Attention-Based Convolutional Neural Network for Intrusion Detection in IoT Networks, Momand, Asadullah, Jan, Sana Ullah, Ramzan, Naeem, Wireless Personal Communications, ISSN 0929-6212, Issue 4, Volume 136, 2024.
Digital Object Identifier: 10.1007/s11277-024-11260-7 [CrossRef]
[7] GNSS jamming detection using attention-based mutual information feature selection, Reda, Ali, Mekkawy, Tamer, Discover Applied Sciences, ISSN 3004-9261, Issue 4, Volume 6, 2024.
Digital Object Identifier: 10.1007/s42452-024-05792-7 [CrossRef]
[8] Learning-based intrusion detection for high-dimensional imbalanced traffic, Gu, Yuheng, Yang, Yu, Yan, Yu, Shen, Fang, Gao, Minna, Computer Communications, ISSN 0140-3664, Issue , 2023.
Digital Object Identifier: 10.1016/j.comcom.2023.10.018 [CrossRef]
[9] Network Intrusion Detection Method Based on Multi-Scale CNN in Internet of Things, Yin, Xiuye, Chen, Liyong, Sun, Le, Mobile Information Systems, ISSN 1875-905X, Issue , 2022.
Digital Object Identifier: 10.1155/2022/8124831 [CrossRef]
[10] Network traffic prediction model based on linear and nonlinear model combination, Lian, Lian, ETRI Journal, ISSN 1225-6463, Issue 3, Volume 46, 2024.
Digital Object Identifier: 10.4218/etrij.2023-0136 [CrossRef]
[11] Intrusion Detection in IoT Environment Using Stochastic Gradient Descent with Warm Restarts and Gated Recurrent Unit, Krishnamurthy, Revatthy, Alabdeli, Haider, N, Rajani, Ramesh, Vankudoth, Vijay Kumar, Bura, 2024 International Conference on Data Science and Network Security (ICDSNS), ISBN 979-8-3503-7311-0, 2024.
Digital Object Identifier: 10.1109/ICDSNS62112.2024.10691232 [CrossRef]
[12] Mayfly Optimization Algorithm with Bidirectional Long-Short Term Memory for Intrusion Detection System in Internet of Things, E Vadakkethil, Sanjaikanth, Polimetla, Kiran, Alsalami, Zaid, Kumar Pareek, Piyush, Kumar, Deepak, 2024 Third International Conference on Distributed Computing and Electrical Circuits and Electronics (ICDCECE), ISBN 979-8-3503-1860-9, 2024.
Digital Object Identifier: 10.1109/ICDCECE60827.2024.10549401 [CrossRef]
Disclaimer: All information displayed above was retrieved by using remote connections to respective databases. For the best user experience, we update all data by using background processes, and use caches in order to reduce the load on the servers we retrieve the information from. As we have no control on the availability of the database servers and sometimes the Internet connectivity may be affected, we do not guarantee the information is correct or complete. For the most accurate data, please always consult the database sites directly. Some external links require authentication or an institutional subscription.
Web of Science® is a registered trademark of Clarivate Analytics, Scopus® is a registered trademark of Elsevier B.V., other product names, company names, brand names, trademarks and logos are the property of their respective owners.
Faculty of Electrical Engineering and Computer Science
Stefan cel Mare University of Suceava, Romania
All rights reserved: Advances in Electrical and Computer Engineering is a registered trademark of the Stefan cel Mare University of Suceava. No part of this publication may be reproduced, stored in a retrieval system, photocopied, recorded or archived, without the written permission from the Editor. When authors submit their papers for publication, they agree that the copyright for their article be transferred to the Faculty of Electrical Engineering and Computer Science, Stefan cel Mare University of Suceava, Romania, if and only if the articles are accepted for publication. The copyright covers the exclusive rights to reproduce and distribute the article, including reprints and translations.
Permission for other use: The copyright owner's consent does not extend to copying for general distribution, for promotion, for creating new works, or for resale. Specific written permission must be obtained from the Editor for such copying. Direct linking to files hosted on this website is strictly prohibited.
Disclaimer: Whilst every effort is made by the publishers and editorial board to see that no inaccurate or misleading data, opinions or statements appear in this journal, they wish to make it clear that all information and opinions formulated in the articles, as well as linguistic accuracy, are the sole responsibility of the author.