2/2019 - 1 | View TOC | « Previous Article | Next Article » |
A Method Based on Lorenz Disturbance and Variational Mode Decomposition for Wind Speed PredictionZHANG, Y.![]() ![]() ![]() ![]() ![]() ![]() ![]() ![]() ![]() ![]() ![]() ![]() |
Extra paper information in ![]() ![]() ![]() |
Click to see author's profile in ![]() ![]() ![]() |
Download PDF ![]() |
Author keywords
wind speed prediction, atmospheric dynamics system, Lorenz system, artificial neural network
References keywords
wind(27), speed(22), energy(18), forecasting(16), model(13), prediction(11), neural(11), novel(8), systems(7), renewable(7)
Blue keywords are present in both the references section and the paper title.
About this article
Date of Publication: 2019-05-31
Volume 19, Issue 2, Year 2019, On page(s): 3 - 12
ISSN: 1582-7445, e-ISSN: 1844-7600
Digital Object Identifier: 10.4316/AECE.2019.02001
Web of Science Accession Number: 000475806300001
SCOPUS ID: 85066296866
Abstract
Wind power is one of the most promising means of power generation. But the time-varying of wind speed is the most fundamental problem for power generation control system. Therefore, accurate wind speed prediction becomes particularly important. However, traditional wind speed predictions often lack consideration of the influence of atmospheric dynamic system. And few papers have introduced VMD method into the field of wind speed prediction. Thus, combined with four neural networks, this paper develops a wind speed prediction method based on Lorenz system and VMD, obtains LD-VMD-Elman wind speed prediction model. Simulation results show that: 1) As for wind speed prediction, Elman neural network has higher prediction accuracy and smaller error. 2) The models which added Lorenz disturbance can describe the actual physical movement of wind more accurately. 3) VMD can abstract the changing rules of different wind speed frequencies to improve the prediction effect. This paper makes up for the lack of consideration of atmospheric dynamic system. The Lorenz equation is used to describe the atmospheric dynamic system, which provides a new thought for wind speed prediction. The LD-VMD-Elman model significantly improves the accuracy of wind speed prediction and contribute to the power dispatch planning. |
References | | | Cited By |
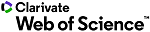
Web of Science® Times Cited: 12 [View]
View record in Web of Science® [View]
View Related Records® [View]
Updated 1 week, 6 days ago
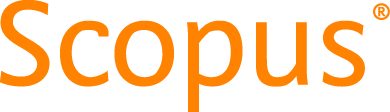
SCOPUS® Times Cited: 18
View record in SCOPUS® [Free preview]
View citations in SCOPUS® [Free preview]
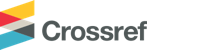
[1] A hybrid prediction model for forecasting wind energy resources, Zhang, Yagang, Pan, Guifang, Environmental Science and Pollution Research, ISSN 0944-1344, Issue 16, Volume 27, 2020.
Digital Object Identifier: 10.1007/s11356-020-08452-6 [CrossRef]
[2] Examining the spatiotemporal variations and inequality of China’s provincial CO2 emissions, Wu, Xiaokun, Hu, Fei, Han, Jingyi, Zhang, Yagang, Environmental Science and Pollution Research, ISSN 0944-1344, Issue 14, Volume 27, 2020.
Digital Object Identifier: 10.1007/s11356-020-08181-w [CrossRef]
[3] A new prediction method based on VMD-PRBF-ARMA-E model considering wind speed characteristic, Zhang, Yagang, Zhao, Yuan, Kong, Chunhui, Chen, Bing, Energy Conversion and Management, ISSN 0196-8904, Issue , 2020.
Digital Object Identifier: 10.1016/j.enconman.2019.112254 [CrossRef]
[4] Enhancing rock and soil hazard monitoring in open-pit mining operations through ultra-short-term wind speed prediction, Sun, Pengxiang, Wang, Juan, Yan, Zhenguo, Frontiers in Earth Science, ISSN 2296-6463, Issue , 2024.
Digital Object Identifier: 10.3389/feart.2023.1297690 [CrossRef]
[5] Short-term wind speed prediction model based on GA-ANN improved by VMD, Zhang, Yagang, Pan, Guifang, Chen, Bing, Han, Jingyi, Zhao, Yuan, Zhang, Chenhong, Renewable Energy, ISSN 0960-1481, Issue , 2020.
Digital Object Identifier: 10.1016/j.renene.2019.12.047 [CrossRef]
[6] One-Month-Ahead Wind Speed Forecasting Using Hybrid AI Model for Coastal Locations, Bou-Rabee, Mohammed, Lodi, Kaif Ahmed, Ali, Mohammad, Faizan Ansari, Mohd, Tariq, Mohd, Anwar Sulaiman, Shaharin, IEEE Access, ISSN 2169-3536, Issue , 2020.
Digital Object Identifier: 10.1109/ACCESS.2020.3028259 [CrossRef]
[7] Intelligent Transportation Application and Analysis for Multi-Sensor Information Fusion of Internet of Things, Li, Ang, Zheng, Baoyu, Li, Lei, IEEE Sensors Journal, ISSN 1530-437X, Issue 22, Volume 21, 2021.
Digital Object Identifier: 10.1109/JSEN.2020.3034911 [CrossRef]
[8] An optimized complementary prediction method based on data feature extraction for wind speed forecasting, Wang, Jujie, Gao, Dongming, Zhuang, Zhenzhen, Wu, Jie, Sustainable Energy Technologies and Assessments, ISSN 2213-1388, Issue , 2022.
Digital Object Identifier: 10.1016/j.seta.2022.102068 [CrossRef]
[9] Performance Evaluation of a New BP Algorithm for a Modified Artificial Neural Network, Panda, Sashmita, Panda, Ganapati, Neural Processing Letters, ISSN 1370-4621, Issue 2, Volume 51, 2020.
Digital Object Identifier: 10.1007/s11063-019-10172-z [CrossRef]
[10] Wind Speed Prediction Research Considering Wind Speed Ramp and Residual Distribution, Zhang, Yagang, Gao, Shuang, Han, Jingyi, Ban, Minghui, IEEE Access, ISSN 2169-3536, Issue , 2019.
Digital Object Identifier: 10.1109/ACCESS.2019.2940897 [CrossRef]
[11] Wind Speed Prediction Based on Gradient Boosting Decision Tree, Fan, Yuxiang, Lei, Weixuan, 2022 International Conference on Big Data, Information and Computer Network (BDICN), ISBN 978-1-6654-8476-3, 2022.
Digital Object Identifier: 10.1109/BDICN55575.2022.00025 [CrossRef]
Disclaimer: All information displayed above was retrieved by using remote connections to respective databases. For the best user experience, we update all data by using background processes, and use caches in order to reduce the load on the servers we retrieve the information from. As we have no control on the availability of the database servers and sometimes the Internet connectivity may be affected, we do not guarantee the information is correct or complete. For the most accurate data, please always consult the database sites directly. Some external links require authentication or an institutional subscription.
Web of Science® is a registered trademark of Clarivate Analytics, Scopus® is a registered trademark of Elsevier B.V., other product names, company names, brand names, trademarks and logos are the property of their respective owners.
Faculty of Electrical Engineering and Computer Science
Stefan cel Mare University of Suceava, Romania
All rights reserved: Advances in Electrical and Computer Engineering is a registered trademark of the Stefan cel Mare University of Suceava. No part of this publication may be reproduced, stored in a retrieval system, photocopied, recorded or archived, without the written permission from the Editor. When authors submit their papers for publication, they agree that the copyright for their article be transferred to the Faculty of Electrical Engineering and Computer Science, Stefan cel Mare University of Suceava, Romania, if and only if the articles are accepted for publication. The copyright covers the exclusive rights to reproduce and distribute the article, including reprints and translations.
Permission for other use: The copyright owner's consent does not extend to copying for general distribution, for promotion, for creating new works, or for resale. Specific written permission must be obtained from the Editor for such copying. Direct linking to files hosted on this website is strictly prohibited.
Disclaimer: Whilst every effort is made by the publishers and editorial board to see that no inaccurate or misleading data, opinions or statements appear in this journal, they wish to make it clear that all information and opinions formulated in the articles, as well as linguistic accuracy, are the sole responsibility of the author.