2/2020 - 13 |
Convolutional Neural Network Based Prediction of Conversion from Mild Cognitive Impairment to Alzheimer's Disease: A Technique using Hippocampus Extracted from MRIMUKHTAR, G.![]() ![]() ![]() ![]() ![]() ![]() |
View the paper record and citations in ![]() |
Click to see author's profile in ![]() ![]() ![]() |
Download PDF ![]() |
Author keywords
artificial neural networks, computer aided diagnosis, image analysis, image classification, pattern recognition
References keywords
alzheimer(35), disease(29), cognitive(13), prediction(12), mild(12), impairment(12), conversion(11), classification(10), brain(10), neuroimage(9)
Blue keywords are present in both the references section and the paper title.
About this article
Date of Publication: 2020-05-31
Volume 20, Issue 2, Year 2020, On page(s): 113 - 122
ISSN: 1582-7445, e-ISSN: 1844-7600
Digital Object Identifier: 10.4316/AECE.2020.02013
Web of Science Accession Number: 000537943500013
SCOPUS ID: 85087437924
Abstract
Alzheimer's disease (AD) is an irreversible neurodegenerative disorder. Mild Cognitive Impairment (MCI) is a prodromal stage of AD and its identification is very crucial for early treatment. MCI to AD conversion is of imperative concern in current Alzheimer's research. In this study, we have investigated the conversion from MCI to AD using different types of features. The impact of structural changes in entire brain tissues captured through MRI, genetics, neuropsychological assessment scores and their combination are investigated. Computational cost can be significantly reduced by examining only the hippocampi region, atrophy of which is visible in the earliest stages of the disease. We proposed a CNN based deep learning approach for the prediction of conversion from MCI to AD using above mentioned features. Highest accuracy is achieved when left hippocampus is used as a region of interest (ROI). The proposed technique outperforms the other state of the art methods, while maintaining a low computational cost. The main contribution of the research lies in the fact that only a single slice based small region of MRI is used resulting in an outstanding performance. The accuracy, sensitivity and specificity achieved are 94%, 92% and 96% respectively. |
References | | | Cited By |
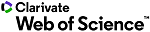
Web of Science® Times Cited: 5 [View]
View record in Web of Science® [View]
View Related Records® [View]
Updated today
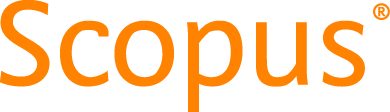
SCOPUS® Times Cited: 10
View record in SCOPUS® [Free preview]
View citations in SCOPUS® [Free preview]
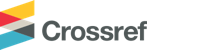
[1] AI-based tool for early detection of Alzheimer's disease, Ul Rehman, Shafiq, Tarek, Noha, Magdy, Caroline, Kamel, Mohammed, Abdelhalim, Mohammed, Melek, Alaa, N. Mahmoud, Lamees, Sadek, Ibrahim, Heliyon, ISSN 2405-8440, Issue 8, Volume 10, 2024.
Digital Object Identifier: 10.1016/j.heliyon.2024.e29375 [CrossRef]
[2] Prediction of Alzheimer’s Disease Progression Based on Magnetic Resonance Imaging, Zhou, Ying, Song, Zeyu, Han, Xiao, Li, Hanjun, Tang, Xiaoying, ACS Chemical Neuroscience, ISSN 1948-7193, Issue 22, Volume 12, 2021.
Digital Object Identifier: 10.1021/acschemneuro.1c00472 [CrossRef]
[3] Neuropsychological detection and prediction using machine learning algorithms: a comprehensive review, Shah, Manan, Shandilya, Ananya, Patel, Kirtan, Mehta, Manya, Sanghavi, Jay, Pandya, Aum, Intelligent Medicine, ISSN 2667-1026, 2023.
Digital Object Identifier: 10.1016/j.imed.2023.04.003 [CrossRef]
[4] Predictive modelling of brain disorders with magnetic resonance imaging: A systematic review of modelling practices, transparency, and interpretability in the use of convolutional neural networks, O'Connell, Shane, Cannon, Dara M., Broin, Pilib Ó., Human Brain Mapping, ISSN 1065-9471, Issue 18, Volume 44, 2023.
Digital Object Identifier: 10.1002/hbm.26521 [CrossRef]
[5] A Deep Longitudinal Model for Mild Cognitive Impairment to Alzheimer’s Disease Conversion Prediction in Low-Income Countries, Akhtar, Adeem, Minhas, Sidra, Sabahat, Nosheen, Khanum, Aasia, Karras, Dimitrios A., Applied Computational Intelligence and Soft Computing, ISSN 1687-9732, Issue , 2022.
Digital Object Identifier: 10.1155/2022/1419310 [CrossRef]
[6] Alzheimer Disease Detection Using MRI: Deep Learning Review, Saikia, Pallavi, Kalita, Sanjib Kumar, SN Computer Science, ISSN 2661-8907, Issue 5, Volume 5, 2024.
Digital Object Identifier: 10.1007/s42979-024-02868-4 [CrossRef]
[7] Robust 2-bit Quantization of Weights in Neural Network Modeled by Laplacian Distribution, PERIC, Z., DENIC, B., DINCIC, M., NIKOLIC, J., Advances in Electrical and Computer Engineering, ISSN 1582-7445, Issue 3, Volume 21, 2021.
Digital Object Identifier: 10.4316/AECE.2021.03001 [CrossRef] [Full text]
[8] Analysis of 32-bit Fixed Point Quantizer in the Wide Variance Range for the Laplacian Source, Peric, Zoran, Jovanovic, Aleksandra, Dincic, Milan, Savic, Milan, Vucic, Nikola, Nikolic, Anastasija, 2021 15th International Conference on Advanced Technologies, Systems and Services in Telecommunications (TELSIKS), ISBN 978-1-6654-4442-2, 2021.
Digital Object Identifier: 10.1109/TELSIKS52058.2021.9606251 [CrossRef]
Disclaimer: All information displayed above was retrieved by using remote connections to respective databases. For the best user experience, we update all data by using background processes, and use caches in order to reduce the load on the servers we retrieve the information from. As we have no control on the availability of the database servers and sometimes the Internet connectivity may be affected, we do not guarantee the information is correct or complete. For the most accurate data, please always consult the database sites directly. Some external links require authentication or an institutional subscription.
Web of Science® is a registered trademark of Clarivate Analytics, Scopus® is a registered trademark of Elsevier B.V., other product names, company names, brand names, trademarks and logos are the property of their respective owners.
Faculty of Electrical Engineering and Computer Science
Stefan cel Mare University of Suceava, Romania
All rights reserved: Advances in Electrical and Computer Engineering is a registered trademark of the Stefan cel Mare University of Suceava. No part of this publication may be reproduced, stored in a retrieval system, photocopied, recorded or archived, without the written permission from the Editor. When authors submit their papers for publication, they agree that the copyright for their article be transferred to the Faculty of Electrical Engineering and Computer Science, Stefan cel Mare University of Suceava, Romania, if and only if the articles are accepted for publication. The copyright covers the exclusive rights to reproduce and distribute the article, including reprints and translations.
Permission for other use: The copyright owner's consent does not extend to copying for general distribution, for promotion, for creating new works, or for resale. Specific written permission must be obtained from the Editor for such copying. Direct linking to files hosted on this website is strictly prohibited.
Disclaimer: Whilst every effort is made by the publishers and editorial board to see that no inaccurate or misleading data, opinions or statements appear in this journal, they wish to make it clear that all information and opinions formulated in the articles, as well as linguistic accuracy, are the sole responsibility of the author.