3/2017 - 14 | View TOC | « Previous Article | Next Article » |
Wind Speed Prediction with Wavelet Time Series Based on Lorenz DisturbanceZHANG, Y.![]() ![]() ![]() ![]() ![]() ![]() ![]() ![]() ![]() ![]() ![]() ![]() |
View the paper record and citations in ![]() |
Click to see author's profile in ![]() ![]() ![]() |
Download PDF ![]() |
Author keywords
ARMA model, Lorenz system, renewable energy, wavelet decomposition, wind speed prediction
References keywords
wind(14), energy(10), speed(8), prediction(8), time(6), power(6), systems(5), series(5), forecasting(5), models(4)
Blue keywords are present in both the references section and the paper title.
About this article
Date of Publication: 2017-08-31
Volume 17, Issue 3, Year 2017, On page(s): 107 - 114
ISSN: 1582-7445, e-ISSN: 1844-7600
Digital Object Identifier: 10.4316/AECE.2017.03014
Web of Science Accession Number: 000410369500014
SCOPUS ID: 85030118150
Abstract
Due to the sustainable and pollution-free characteristics, wind energy has been one of the fastest growing renewable energy sources. However, the intermittent and random fluctuation of wind speed presents many challenges for reliable wind power integration and normal operation of wind farm. Accurate wind speed prediction is the key to ensure the safe operation of power system and to develop wind energy resources. Therefore, this paper has presented a wavelet time series wind speed prediction model based on Lorenz disturbance. Therefore, in this paper, combined with the atmospheric dynamical system, a wavelet-time series improved wind speed prediction model based on Lorenz disturbance is proposed and the wind turbines of different climate types in Spain and China are used to simulate the disturbances of Lorenz equations with different initial values. The prediction results show that the improved model can effectively correct the preliminary prediction of wind speed, improving the prediction. In a word, the research work in this paper will be helpful to arrange the electric power dispatching plan and ensure the normal operation of the wind farm. |
References | | | Cited By |
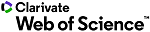
Web of Science® Times Cited: 27 [View]
View record in Web of Science® [View]
View Related Records® [View]
Updated 3 days, 1 hour
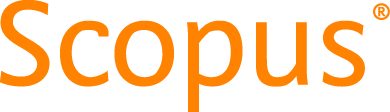
SCOPUS® Times Cited: 28
View record in SCOPUS® [Free preview]
View citations in SCOPUS® [Free preview]
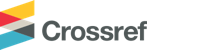
[1] Multi-sensors based condition monitoring of rotary machines: An approach of multidimensional time-series analysis, Wang, Teng, Lu, Guoliang, Yan, Peng, Measurement, ISSN 0263-2241, Issue , 2019.
Digital Object Identifier: 10.1016/j.measurement.2018.10.089 [CrossRef]
[2] Wind speed prediction research with EMD-BP based on Lorenz disturbance, Zhang, Yagang, Pan, Guifang, Zhang, Chenhong, Zhao, Yuan, Journal of Electrical Engineering, ISSN 1339-309X, Issue 3, Volume 70, 2019.
Digital Object Identifier: 10.2478/jee-2019-0028 [CrossRef]
[3] A domain association hierarchical decomposition optimization method for cab vibration control of commercial vehicles, He, Shuilong, Tang, Tao, Ye, Mingsong, Xu, Enyong, Deng, Jucai, Tang, Rongjiang, Measurement, ISSN 0263-2241, Issue , 2019.
Digital Object Identifier: 10.1016/j.measurement.2019.02.020 [CrossRef]
[4] A Novel Hybrid Model for Wind Speed Prediction Based on VMD and Neural Network Considering Atmospheric Uncertainties, Zhang, Yagang, Zhao, Yuan, Gao, Shuang, IEEE Access, ISSN 2169-3536, Issue , 2019.
Digital Object Identifier: 10.1109/ACCESS.2019.2915582 [CrossRef]
[5] Wind Speed Prediction of IPSO-BP Neural Network Based on Lorenz Disturbance, Zhang, Yagang, Chen, Bing, Zhao, Yuan, Pan, Guifang, IEEE Access, ISSN 2169-3536, Issue , 2018.
Digital Object Identifier: 10.1109/ACCESS.2018.2869981 [CrossRef]
[6] A sparse stacked denoising autoencoder with optimized transfer learning applied to the fault diagnosis of rolling bearings, Sun, Meidi, Wang, Hui, Liu, Ping, Huang, Shoudao, Fan, Peng, Measurement, ISSN 0263-2241, Issue , 2019.
Digital Object Identifier: 10.1016/j.measurement.2019.06.029 [CrossRef]
[7] Short-term wind speed prediction model based on GA-ANN improved by VMD, Zhang, Yagang, Pan, Guifang, Chen, Bing, Han, Jingyi, Zhao, Yuan, Zhang, Chenhong, Renewable Energy, ISSN 0960-1481, Issue , 2020.
Digital Object Identifier: 10.1016/j.renene.2019.12.047 [CrossRef]
[8] Evaluation of neural network-based methodologies for wind speed forecasting, Samet, Haidar, Reisi, Mohammad, Marzbani, Fatemeh, Computers & Electrical Engineering, ISSN 0045-7906, Issue , 2019.
Digital Object Identifier: 10.1016/j.compeleceng.2019.07.024 [CrossRef]
[9] Short-Term Canyon Wind Speed Prediction Based on CNN—GRU Transfer Learning, Ji, Lipeng, Fu, Chenqi, Ju, Zheng, Shi, Yicheng, Wu, Shun, Tao, Li, Atmosphere, ISSN 2073-4433, Issue 5, Volume 13, 2022.
Digital Object Identifier: 10.3390/atmos13050813 [CrossRef]
[10] Detection of Cracks and damage in wind turbine blades using artificial intelligence-based image analytics, Reddy, Abhishek, Indragandhi, V., Ravi, Logesh, Subramaniyaswamy, V., Measurement, ISSN 0263-2241, Issue , 2019.
Digital Object Identifier: 10.1016/j.measurement.2019.07.051 [CrossRef]
[11] Application and analysis of hydraulic wind power generation technology, Liu, Keyi, Chen, Wei, Chen, Gexin, Dai, Dandan, Ai, Chao, Zhang, Xinwang, Wang, Xin, Energy Strategy Reviews, ISSN 2211-467X, Issue , 2023.
Digital Object Identifier: 10.1016/j.esr.2023.101117 [CrossRef]
[12] An efficient short-term wind speed prediction model based on cross-channel data integration and attention mechanisms, Yu, Enbo, Xu, Guoji, Han, Yan, Li, Yongle, Energy, ISSN 0360-5442, Issue , 2022.
Digital Object Identifier: 10.1016/j.energy.2022.124569 [CrossRef]
[13] Wind speed prediction with RBF neural network based on PCA and ICA, Zhang, Yagang, Zhang, Chenhong, Zhao, Yuan, Gao, Shuang, Journal of Electrical Engineering, ISSN 1339-309X, Issue 2, Volume 69, 2018.
Digital Object Identifier: 10.2478/jee-2018-0018 [CrossRef]
[14] A three-dimensional geometric features-based SCA algorithm for compound faults diagnosis, Hao, Yansong, Song, Liuyang, Cui, Lingli, Wang, Huaqing, Measurement, ISSN 0263-2241, Issue , 2019.
Digital Object Identifier: 10.1016/j.measurement.2018.10.098 [CrossRef]
[15] A novel hybrid model based on VMD-WT and PCA-BP-RBF neural network for short-term wind speed forecasting, Zhang, Yagang, Chen, Bing, Pan, Guifang, Zhao, Yuan, Energy Conversion and Management, ISSN 0196-8904, Issue , 2019.
Digital Object Identifier: 10.1016/j.enconman.2019.05.005 [CrossRef]
[16] A Validity Index for Fuzzy Clustering Based on Bipartite Modularity, Liu, Yongli, Zhang, Xiaoyang, Chen, Jingli, Chao, Hao, Journal of Electrical and Computer Engineering, ISSN 2090-0147, Issue , 2019.
Digital Object Identifier: 10.1155/2019/2719617 [CrossRef]
[17] A Method Based on Lorenz Disturbance and Variational Mode Decomposition for Wind Speed Prediction, ZHANG, Y., GAO, S., BAN, M., SUN, Y., Advances in Electrical and Computer Engineering, ISSN 1582-7445, Issue 2, Volume 19, 2019.
Digital Object Identifier: 10.4316/AECE.2019.02001 [CrossRef] [Full text]
[18] Automatic detection of a wheelset bearing fault using a multi-level empirical wavelet transform, Ding, Jianming, Ding, Chengcheng, Measurement, ISSN 0263-2241, Issue , 2019.
Digital Object Identifier: 10.1016/j.measurement.2018.10.064 [CrossRef]
[19] A novel improved full vector spectrum algorithm and its application in multi-sensor data fusion for hydraulic pumps, Yu, He, Li, Hongru, Li, Yaolong, Li, Yifan, Measurement, ISSN 0263-2241, Issue , 2019.
Digital Object Identifier: 10.1016/j.measurement.2018.10.011 [CrossRef]
[20] Bearing fault diagnosis based on Cluster-contraction Stage-wise Orthogonal-Matching-Pursuit, Song, Liu, Yan, Ruqiang, Measurement, ISSN 0263-2241, Issue , 2019.
Digital Object Identifier: 10.1016/j.measurement.2019.03.061 [CrossRef]
[21] Wind Speed Prediction Using Wavelet Decomposition Based on Lorenz Disturbance Model, Zhang, Yagang, Zhang, Chenhong, Gao, Shuang, Wang, Penghui, Xie, Fenglin, Cheng, Penglai, Lei, Shuang, IETE Journal of Research, ISSN 0377-2063, Issue 5, Volume 66, 2020.
Digital Object Identifier: 10.1080/03772063.2018.1512384 [CrossRef]
[22] A new laboratory test method for tire-pavement noise, Ren, Wanyan, Han, Sen, Fwa, Tien Fang, Zhang, Jiahao, He, Zhihao, Measurement, ISSN 0263-2241, Issue , 2019.
Digital Object Identifier: 10.1016/j.measurement.2019.05.096 [CrossRef]
[23] A Rolling Bearing Fault Diagnosis-Optimized Scale-Space Representation for the Empirical Wavelet Transform, Liu, Zechao, Ding, Jianming, Lin, Jianhui, Huang, Yan, Shock and Vibration, ISSN 1070-9622, Issue , 2018.
Digital Object Identifier: 10.1155/2018/2749689 [CrossRef]
[24] States prediction for solar power and wind speed using BBA‐SVM, Li, Zhen‐Long, Xia, Jing, Liu, An, Li, Peng, IET Renewable Power Generation, ISSN 1752-1416, Issue 7, Volume 13, 2019.
Digital Object Identifier: 10.1049/iet-rpg.2018.5673 [CrossRef]
[25] Wind Speed Interval Prediction Based on Lorenz Disturbance Distribution, Zhang, Yagang, Zhao, Yuan, Pan, Guifang, Zhang, Jinfang, IEEE Transactions on Sustainable Energy, ISSN 1949-3029, Issue 2, Volume 11, 2020.
Digital Object Identifier: 10.1109/TSTE.2019.2907699 [CrossRef]
[26] Short-term wind speed prediction model of VMD-FOAGRNN based on Lorenz disturbance, Pan, Guifang, Han, Jingyi, Zhang, Yagang, 2019 IEEE Sustainable Power and Energy Conference (iSPEC), ISBN 978-1-7281-4930-1, 2019.
Digital Object Identifier: 10.1109/iSPEC48194.2019.8974883 [CrossRef]
Disclaimer: All information displayed above was retrieved by using remote connections to respective databases. For the best user experience, we update all data by using background processes, and use caches in order to reduce the load on the servers we retrieve the information from. As we have no control on the availability of the database servers and sometimes the Internet connectivity may be affected, we do not guarantee the information is correct or complete. For the most accurate data, please always consult the database sites directly. Some external links require authentication or an institutional subscription.
Web of Science® is a registered trademark of Clarivate Analytics, Scopus® is a registered trademark of Elsevier B.V., other product names, company names, brand names, trademarks and logos are the property of their respective owners.
Faculty of Electrical Engineering and Computer Science
Stefan cel Mare University of Suceava, Romania
All rights reserved: Advances in Electrical and Computer Engineering is a registered trademark of the Stefan cel Mare University of Suceava. No part of this publication may be reproduced, stored in a retrieval system, photocopied, recorded or archived, without the written permission from the Editor. When authors submit their papers for publication, they agree that the copyright for their article be transferred to the Faculty of Electrical Engineering and Computer Science, Stefan cel Mare University of Suceava, Romania, if and only if the articles are accepted for publication. The copyright covers the exclusive rights to reproduce and distribute the article, including reprints and translations.
Permission for other use: The copyright owner's consent does not extend to copying for general distribution, for promotion, for creating new works, or for resale. Specific written permission must be obtained from the Editor for such copying. Direct linking to files hosted on this website is strictly prohibited.
Disclaimer: Whilst every effort is made by the publishers and editorial board to see that no inaccurate or misleading data, opinions or statements appear in this journal, they wish to make it clear that all information and opinions formulated in the articles, as well as linguistic accuracy, are the sole responsibility of the author.