2/2023 - 6 |
Epilepsy Seizure Prediction from EEG Signal Using Machine Learning TechniquesSIDAOUI, B.![]() ![]() ![]() ![]() ![]() ![]() |
Extra paper information in ![]() ![]() ![]() |
Click to see author's profile in ![]() ![]() ![]() |
Download PDF ![]() |
Author keywords
epilepsy seizure, EEG, prediction, Convolutional Neural Network, SVM
References keywords
detection(13), seizure(10), neural(9), learning(9), epilepsy(7), epileptic(6), deep(6), vector(5), support(5), networks(5)
Blue keywords are present in both the references section and the paper title.
About this article
Date of Publication: 2023-05-31
Volume 23, Issue 2, Year 2023, On page(s): 47 - 54
ISSN: 1582-7445, e-ISSN: 1844-7600
Digital Object Identifier: 10.4316/AECE.2023.02006
Web of Science Accession Number: 001009953400006
SCOPUS ID: 85164319612
Abstract
Automatic seizure prediction is an important task to help epilepsy patients and epilepsy specialists. In addition, measuring electrical activity in different brain parts is an important step before any prediction. The best tool for recording electrical activity is electroencephalography (EEG), which uses electrodes placed on the head. This paper examines the performance of the convolutional neural network (CNN) architectures and support vector machine (SVM) method for predicting epileptic seizure activity using rich information recorded in the signal of EEG segments. The proposed approach is based on 22 features extracted from different EEG segments to produce a representative dataset. SVM classification models and two CNN architectures are proposed to predict ongoing seizures and different states of epilepsy patients. Two CNN architectures are presented: the first is trained with a dataset of features extracted from the EEG signal, and the second is trained with a dataset of Scalogram images from the EEG signal, whose purpose is to predict the imminence of an epileptic seizure in patients. A dataset of 6 patients is used to predict all states of epilepsy patients. Both CNN architectures and binary SVM classifiers achieve a classification rate above 98%. |
References | | | Cited By |
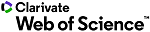
Web of Science® Times Cited: 4 [View]
View record in Web of Science® [View]
View Related Records® [View]
Updated 1 week, 4 days ago
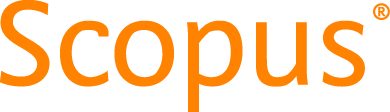
SCOPUS® Times Cited: 4
View record in SCOPUS® [Free preview]
View citations in SCOPUS® [Free preview]
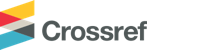
[1] Detection Method of Epileptic Seizures Using a Neural Network Model Based on Multimodal Dual-Stream Networks, Wang, Baiyang, Xu, Yidong, Peng, Siyu, Wang, Hongjun, Li, Fang, Sensors, ISSN 1424-8220, Issue 11, Volume 24, 2024.
Digital Object Identifier: 10.3390/s24113360 [CrossRef]
[2] Distance optimization KNN and EMD based lightweight hardware IP core design for EEG epilepsy detection, Chen, Xuanxu, Zhang, Yuejun, Ai, Guangpeng, Wang, Lixun, Zhang, Huihong, Li, Xiangyu, Wang, Pengjun, Microelectronics Journal, ISSN 1879-2391, Issue , 2024.
Digital Object Identifier: 10.1016/j.mejo.2024.106335 [CrossRef]
[3] Effects of Sampling Length and Overlap Ratio on EEG Mental Arithmetic Task Performance: A Comparative Study, Oran, Samet, Yıldırım, Esen, Gazi University Journal of Science, ISSN 2147-1762, Issue 4, Volume 37, 2024.
Digital Object Identifier: 10.35378/gujs.1413191 [CrossRef]
[4] Predicting antiseizure medication response in newly diagnosed epilepsy using quantitative EEG and machine learning, Lee, Gha-Hyun, Sung, Sang Min, Choi, Kwang-Dong, Kim, Jiyoung, Cho, Jae Wook, Kim, Sang Ho, Seizure: European Journal of Epilepsy, ISSN 1059-1311, Issue , 2025.
Digital Object Identifier: 10.1016/j.seizure.2025.05.007 [CrossRef]
Disclaimer: All information displayed above was retrieved by using remote connections to respective databases. For the best user experience, we update all data by using background processes, and use caches in order to reduce the load on the servers we retrieve the information from. As we have no control on the availability of the database servers and sometimes the Internet connectivity may be affected, we do not guarantee the information is correct or complete. For the most accurate data, please always consult the database sites directly. Some external links require authentication or an institutional subscription.
Web of Science® is a registered trademark of Clarivate Analytics, Scopus® is a registered trademark of Elsevier B.V., other product names, company names, brand names, trademarks and logos are the property of their respective owners.
Faculty of Electrical Engineering and Computer Science
Stefan cel Mare University of Suceava, Romania
All rights reserved: Advances in Electrical and Computer Engineering is a registered trademark of the Stefan cel Mare University of Suceava. No part of this publication may be reproduced, stored in a retrieval system, photocopied, recorded or archived, without the written permission from the Editor. When authors submit their papers for publication, they agree that the copyright for their article be transferred to the Faculty of Electrical Engineering and Computer Science, Stefan cel Mare University of Suceava, Romania, if and only if the articles are accepted for publication. The copyright covers the exclusive rights to reproduce and distribute the article, including reprints and translations.
Permission for other use: The copyright owner's consent does not extend to copying for general distribution, for promotion, for creating new works, or for resale. Specific written permission must be obtained from the Editor for such copying. Direct linking to files hosted on this website is strictly prohibited.
Disclaimer: Whilst every effort is made by the publishers and editorial board to see that no inaccurate or misleading data, opinions or statements appear in this journal, they wish to make it clear that all information and opinions formulated in the articles, as well as linguistic accuracy, are the sole responsibility of the author.