3/2010 - 6 |
A New MLP Approach for the Detection of the Incipient Bearing DamageSENGULER, T.![]() ![]() ![]() ![]() ![]() ![]() ![]() ![]() ![]() |
Extra paper information in ![]() ![]() ![]() |
Click to see author's profile in ![]() ![]() ![]() |
Download PDF ![]() |
Author keywords
bearing damage, vibration analysis, MLP neural networks, feature extraction, condition monitoring
References keywords
neural(16), networks(10), bearing(8), applications(7), signal(6), processing(6), artificial(6), monitoring(5), electric(5), condition(5)
Blue keywords are present in both the references section and the paper title.
About this article
Date of Publication: 2010-08-31
Volume 10, Issue 3, Year 2010, On page(s): 34 - 39
ISSN: 1582-7445, e-ISSN: 1844-7600
Digital Object Identifier: 10.4316/AECE.2010.03006
Web of Science Accession Number: 000281805600006
SCOPUS ID: 77956621055
Abstract
In this study, it is aimed to track the aging trend of the incipient bearing damage in an induction motor which is subjected to an accelerated aging process. For this purpose, a new Multilayer perceptron (MLP) neural network approach is introduced. The input signals are extracted from power spectral densities (PSD) of the vibration signals taken from a 5-HP induction motor. Principal component analysis (PCA) has been applied to select the best possible feature vectors as a dimensionality reduction purpose. Variance and entropy values are used as the targets of the MLP network. The healthy motor condition was modelled by the MLP network considering all load conditions. The results showed that the incipient bearing damage was clearly extracted by the oscillations of the MLP output error. |
References | | | Cited By |
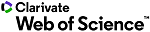
Web of Science® Times Cited: 10 [View]
View record in Web of Science® [View]
View Related Records® [View]
Updated 3 days ago
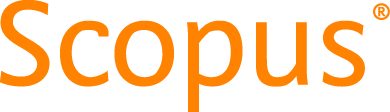
SCOPUS® Times Cited: 13
View record in SCOPUS® [Free preview]
View citations in SCOPUS® [Free preview]
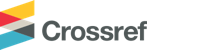
[1] Continuous wavelet transform for ferroresonance detection in power systems, Şengüler, Tayfun, Şeker, Serhat, Electrical Engineering, ISSN 0948-7921, Issue 2, Volume 99, 2017.
Digital Object Identifier: 10.1007/s00202-016-0387-0 [CrossRef]
[2] Fault Detection and Identification Using Deep Learning Algorithms in Induction Motors, Hussain, Majid, Din Memon, Tayab, Hussain, Imtiaz, Ahmed Memon, Zubair, Kumar, Dileep, Computer Modeling in Engineering & Sciences, ISSN 1526-1506, Issue 2, Volume 133, 2022.
Digital Object Identifier: 10.32604/cmes.2022.020583 [CrossRef]
[3] Acoustic Emission-Based Prognostics of Slow Rotating Bearing Using Bayesian Techniques Under Dependent and Independent Samples, Aye, S. A., Heyns, P. S., Applied Artificial Intelligence, ISSN 0883-9514, Issue 6, Volume 29, 2015.
Digital Object Identifier: 10.1080/08839514.2015.1038432 [CrossRef]
[4] Support vector machine classifier for diagnosis in electrical machines: Application to broken bar, Matić, Dragan, Kulić, Filip, Pineda-Sánchez, Manuel, Kamenko, Ilija, Expert Systems with Applications, ISSN 0957-4174, Issue 10, Volume 39, 2012.
Digital Object Identifier: 10.1016/j.eswa.2012.01.214 [CrossRef]
[5] State-Space GMDH Neural Networks for Actuator Robust Fault Diagnosis, MRUGALSKI, M., WITCZAK, M., Advances in Electrical and Computer Engineering, ISSN 1582-7445, Issue 3, Volume 12, 2012.
Digital Object Identifier: 10.4316/aece.2012.03010 [CrossRef] [Full text]
[6] Accurate bearing remaining useful life prediction based on Weibull distribution and artificial neural network, Ben Ali, Jaouher, Chebel-Morello, Brigitte, Saidi, Lotfi, Malinowski, Simon, Fnaiech, Farhat, Mechanical Systems and Signal Processing, ISSN 0888-3270, Issue , 2015.
Digital Object Identifier: 10.1016/j.ymssp.2014.10.014 [CrossRef]
[7] Analysis of statistical features based on start-up current envelope for broken rotor bar fault detection in line start permanent magnet synchronous motor, Mehrjou, Mohammad Rezazadeh, Mariun, Norman, Misron, Norhisam, Radzi, Mohd Amran Mohd, Electrical Engineering, ISSN 0948-7921, Issue 1, Volume 99, 2017.
Digital Object Identifier: 10.1007/s00202-016-0404-3 [CrossRef]
[8] Vibration Based Broken Bar Detection in Induction Machine for Low Load Conditions, MATIC, D., KANOVIC, Z., Advances in Electrical and Computer Engineering, ISSN 1582-7445, Issue 1, Volume 17, 2017.
Digital Object Identifier: 10.4316/AECE.2017.01007 [CrossRef] [Full text]
[9] Classification and Authentication of Induction Motor Faults using Time and Frequency Feature Dependent Probabilistic Neural Network Model, Thakur, Arunava Kabiraj, Mukherjee, Alok, Kundu, Palash Kumar, Das, Arabinda, Journal of The Institution of Engineers (India): Series B, ISSN 2250-2106, Issue 3, Volume 104, 2023.
Digital Object Identifier: 10.1007/s40031-023-00872-5 [CrossRef]
[10] Radial basis function neural network based comparison of dimensionality reduction techniques for effective bearing diagnostics, GS, Vijay, Pai, Srinivasa P, Sriram, NS, Rao, Raj BKN, Proceedings of the Institution of Mechanical Engineers, Part J: Journal of Engineering Tribology, ISSN 1350-6501, Issue 6, Volume 227, 2013.
Digital Object Identifier: 10.1177/1350650112464927 [CrossRef]
[11] Broken rotor bar fault detection in line start permanent magnet synchronous motor using transient current signal, Izadi, Mahdi, Mariun, Norman, Mehrjou, Mohammad Rezazadeh, Abidin Ab Kadir, Mohd Zainal, Misron, Norhisam, Radzi, Mohd Amran Mohd, 2016 IEEE International Conference on Automatic Control and Intelligent Systems (I2CACIS), ISBN 978-1-5090-4186-2, 2016.
Digital Object Identifier: 10.1109/I2CACIS.2016.7885288 [CrossRef]
Disclaimer: All information displayed above was retrieved by using remote connections to respective databases. For the best user experience, we update all data by using background processes, and use caches in order to reduce the load on the servers we retrieve the information from. As we have no control on the availability of the database servers and sometimes the Internet connectivity may be affected, we do not guarantee the information is correct or complete. For the most accurate data, please always consult the database sites directly. Some external links require authentication or an institutional subscription.
Web of Science® is a registered trademark of Clarivate Analytics, Scopus® is a registered trademark of Elsevier B.V., other product names, company names, brand names, trademarks and logos are the property of their respective owners.
Faculty of Electrical Engineering and Computer Science
Stefan cel Mare University of Suceava, Romania
All rights reserved: Advances in Electrical and Computer Engineering is a registered trademark of the Stefan cel Mare University of Suceava. No part of this publication may be reproduced, stored in a retrieval system, photocopied, recorded or archived, without the written permission from the Editor. When authors submit their papers for publication, they agree that the copyright for their article be transferred to the Faculty of Electrical Engineering and Computer Science, Stefan cel Mare University of Suceava, Romania, if and only if the articles are accepted for publication. The copyright covers the exclusive rights to reproduce and distribute the article, including reprints and translations.
Permission for other use: The copyright owner's consent does not extend to copying for general distribution, for promotion, for creating new works, or for resale. Specific written permission must be obtained from the Editor for such copying. Direct linking to files hosted on this website is strictly prohibited.
Disclaimer: Whilst every effort is made by the publishers and editorial board to see that no inaccurate or misleading data, opinions or statements appear in this journal, they wish to make it clear that all information and opinions formulated in the articles, as well as linguistic accuracy, are the sole responsibility of the author.