1/2022 - 7 |
Image Forgery Detection Using Noise and Edge Weighted Local Texture FeaturesASGHAR, K.![]() ![]() ![]() ![]() ![]() ![]() ![]() ![]() ![]() ![]() ![]() ![]() ![]() ![]() ![]() |
View the paper record and citations in ![]() |
Click to see author's profile in ![]() ![]() ![]() |
Download PDF ![]() |
Author keywords
artificial intelligence, forgery, Fourier transforms, machine learning, pattern recognition
References keywords
image(54), detection(35), forgery(28), noise(20), forensics(18), information(15), features(14), security(13), processing(13), multimedia(12)
Blue keywords are present in both the references section and the paper title.
About this article
Date of Publication: 2022-02-28
Volume 22, Issue 1, Year 2022, On page(s): 57 - 69
ISSN: 1582-7445, e-ISSN: 1844-7600
Digital Object Identifier: 10.4316/AECE.2022.01007
Web of Science Accession Number: 000762769600006
SCOPUS ID: 85126766756
Abstract
Image forgery detection is important for sensitive domains such as courts of law. The main challenge is to develop a robust model that is sensitive to tampering traces. Existing techniques perform well on a limited dataset but do not generalize well across the datasets. Moreover, these techniques cannot reliably detect tampering that distorts the texture pattern of the image. The noise patterns remain consistent throughout a digital image if its contents are not altered. Based on this hypothesis, a robust descriptor FFT-DRLBP (Fast Fourier Transformation - Discriminative Robust Local Binary Patterns) is introduced, which first estimates noise patterns using FFT and encodes the discrepancies in noise patterns using DRLBP. Features extracted are passed to Support Vector Machine (SVM) for deciding whether the image is authentic or tampered. Intensive experiments are performed on benchmark datasets to validate the performance of the method. It achieved an accuracy of 99.21% on the combination of two challenging datasets. The comparison shows that it outperforms state-of-the-art methods and is vigorous to image forgery attacks even in the presence of various post-processing operations. The performance of the method is also validated using cross-dataset experiments, which ensures its robustness and generalization. |
References | | | Cited By |
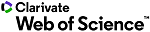
Web of Science® Times Cited: 0
View record in Web of Science® [View]
View Related Records® [View]
Updated today
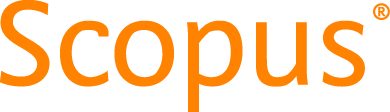
SCOPUS® Times Cited: 1
View record in SCOPUS® [Free preview]
View citations in SCOPUS® [Free preview]
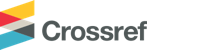
[1] DS‐Net: Dual supervision neural network for image manipulation localization, Dai, Chenwei, Su, Lichao, Wu, Bin, Chen, Jian, IET Image Processing, ISSN 1751-9659, Issue 12, Volume 17, 2023.
Digital Object Identifier: 10.1049/ipr2.12885 [CrossRef]
Disclaimer: All information displayed above was retrieved by using remote connections to respective databases. For the best user experience, we update all data by using background processes, and use caches in order to reduce the load on the servers we retrieve the information from. As we have no control on the availability of the database servers and sometimes the Internet connectivity may be affected, we do not guarantee the information is correct or complete. For the most accurate data, please always consult the database sites directly. Some external links require authentication or an institutional subscription.
Web of Science® is a registered trademark of Clarivate Analytics, Scopus® is a registered trademark of Elsevier B.V., other product names, company names, brand names, trademarks and logos are the property of their respective owners.
Faculty of Electrical Engineering and Computer Science
Stefan cel Mare University of Suceava, Romania
All rights reserved: Advances in Electrical and Computer Engineering is a registered trademark of the Stefan cel Mare University of Suceava. No part of this publication may be reproduced, stored in a retrieval system, photocopied, recorded or archived, without the written permission from the Editor. When authors submit their papers for publication, they agree that the copyright for their article be transferred to the Faculty of Electrical Engineering and Computer Science, Stefan cel Mare University of Suceava, Romania, if and only if the articles are accepted for publication. The copyright covers the exclusive rights to reproduce and distribute the article, including reprints and translations.
Permission for other use: The copyright owner's consent does not extend to copying for general distribution, for promotion, for creating new works, or for resale. Specific written permission must be obtained from the Editor for such copying. Direct linking to files hosted on this website is strictly prohibited.
Disclaimer: Whilst every effort is made by the publishers and editorial board to see that no inaccurate or misleading data, opinions or statements appear in this journal, they wish to make it clear that all information and opinions formulated in the articles, as well as linguistic accuracy, are the sole responsibility of the author.