4/2022 - 9 |
Analog Circuit Fault Classification and Data Reduction Using PCA-ANFIS Technique Aided by K-means Clustering ApproachLAIDANI, I.![]() ![]() ![]() ![]() ![]() ![]() |
Extra paper information in ![]() ![]() ![]() |
Click to see author's profile in ![]() ![]() ![]() |
Download PDF ![]() |
Author keywords
analog integrated circuits, artificial neural networks, fault diagnosis, fuzzy logic, clustering methods
References keywords
analog(18), fault(17), diagnosis(14), circuits(13), circuit(9), fuzzy(7), electronic(6), method(5), classifier(5), approach(5)
Blue keywords are present in both the references section and the paper title.
About this article
Date of Publication: 2022-11-30
Volume 22, Issue 4, Year 2022, On page(s): 73 - 82
ISSN: 1582-7445, e-ISSN: 1844-7600
Digital Object Identifier: 10.4316/AECE.2022.04009
Web of Science Accession Number: 000920289700009
SCOPUS ID: 85150155847
Abstract
The paper work aims to extract effectively the fault feature information of analog integrated circuits and to improve the performance of a fault classification process. Thus, a fault classification method based on principal component analysis (PCA) and adaptive neuro fuzzy inference system classifier (ANFIS) preprocessed by K-means clustering (KMC) is proposed. To effectively extract and select fault features the traditional signal processing based on sampling technique conducts to different signature parameters. A stimulus pulse signal applied to the circuit under test (CUT) allowed us to get a reference output response. Respecting both specific sampling interval and step, the fault free and the faulty output responses are sampled to create amplitude sample features that will serve the fault classification process. The PCA employed for data reduction has lessened the computational complexity and obtaining the optimal features. Thus more than 75% of data volume decreased without loss of original information. The principal components extracted by this reduction data method have been input into ANFIS aided by KMC to obtain the best fault diagnosis results. The experimental results show a score of 100% diagnostic accuracies for the CUTs. Therefore, our approach has achieved best fault classification precision comparing to other research works. |
References | | | Cited By |
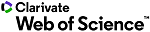
Web of Science® Times Cited: 3 [View]
View record in Web of Science® [View]
View Related Records® [View]
Updated today
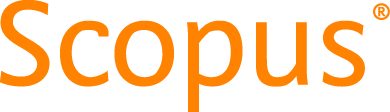
SCOPUS® Times Cited: 3
View record in SCOPUS® [Free preview]
View citations in SCOPUS® [Free preview]
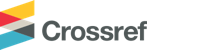
[1] An efficient method for faults diagnosis in analog circuits based on machine learning classifiers, Arabi, Abderrazak, Ayad, Mouloud, Bourouba, Nacerdine, Benziane, Mourad, Griche, Issam, Ghoneim, Sherif S.M., Ali, Enas, Elsisi, Mahmoud, Ghaly, Ramy N.R., Alexandria Engineering Journal, ISSN 1110-0168, Issue , 2023.
Digital Object Identifier: 10.1016/j.aej.2023.06.090 [CrossRef]
[2] Intermittent fault diagnosis for electronics-rich analog circuit systems based on multi-scale enhanced convolution transformer network with novel token fusion strategy, Wang, Shengdong, Liu, Zhenbao, Jia, Zhen, Zhao, Wen, Li, Zihao, Expert Systems with Applications, ISSN 0957-4174, Issue , 2024.
Digital Object Identifier: 10.1016/j.eswa.2023.121964 [CrossRef]
[3] Intermittent fault diagnosis of analog circuit based on enhanced one-dimensional vision transformer and transfer learning strategy, Wang, Shengdong, Liu, Zhenbao, Jia, Zhen, Zhao, Wen, Li, Zihao, Wang, Luyao, Engineering Applications of Artificial Intelligence, ISSN 0952-1976, Issue , 2024.
Digital Object Identifier: 10.1016/j.engappai.2023.107281 [CrossRef]
Disclaimer: All information displayed above was retrieved by using remote connections to respective databases. For the best user experience, we update all data by using background processes, and use caches in order to reduce the load on the servers we retrieve the information from. As we have no control on the availability of the database servers and sometimes the Internet connectivity may be affected, we do not guarantee the information is correct or complete. For the most accurate data, please always consult the database sites directly. Some external links require authentication or an institutional subscription.
Web of Science® is a registered trademark of Clarivate Analytics, Scopus® is a registered trademark of Elsevier B.V., other product names, company names, brand names, trademarks and logos are the property of their respective owners.
Faculty of Electrical Engineering and Computer Science
Stefan cel Mare University of Suceava, Romania
All rights reserved: Advances in Electrical and Computer Engineering is a registered trademark of the Stefan cel Mare University of Suceava. No part of this publication may be reproduced, stored in a retrieval system, photocopied, recorded or archived, without the written permission from the Editor. When authors submit their papers for publication, they agree that the copyright for their article be transferred to the Faculty of Electrical Engineering and Computer Science, Stefan cel Mare University of Suceava, Romania, if and only if the articles are accepted for publication. The copyright covers the exclusive rights to reproduce and distribute the article, including reprints and translations.
Permission for other use: The copyright owner's consent does not extend to copying for general distribution, for promotion, for creating new works, or for resale. Specific written permission must be obtained from the Editor for such copying. Direct linking to files hosted on this website is strictly prohibited.
Disclaimer: Whilst every effort is made by the publishers and editorial board to see that no inaccurate or misleading data, opinions or statements appear in this journal, they wish to make it clear that all information and opinions formulated in the articles, as well as linguistic accuracy, are the sole responsibility of the author.