2/2020 - 15 | View TOC | « Previous Article | Next Article » |
Comparison of Classification Algorithms for Detecting Patient Posture in Expandable Tumor ProsthesesKOCAOGLU, S.![]() ![]() ![]() ![]() ![]() ![]() |
View the paper record and citations in ![]() |
Click to see author's profile in ![]() ![]() ![]() |
Download PDF ![]() |
Author keywords
biomedical measurement, machine learning, prosthetics, supervised learning, support vector machines
References keywords
recognition(19), posture(13), activity(12), wearable(11), detection(9), sensors(8), comput(8), biomed(8), system(7), human(7)
Blue keywords are present in both the references section and the paper title.
About this article
Date of Publication: 2020-05-31
Volume 20, Issue 2, Year 2020, On page(s): 131 - 138
ISSN: 1582-7445, e-ISSN: 1844-7600
Digital Object Identifier: 10.4316/AECE.2020.02015
Web of Science Accession Number: 000537943500015
SCOPUS ID: 85087436149
Abstract
Autonomous tumor prostheses are extended without the need of a clinic and of a medical supervision. It is necessary to make sure that the patient is not standing before extending these prostheses. This study aims to determine the posture of the patient for expandable tumor prostheses by employing oft-used three machine learning-based classification methods through comparing them all with each other. Patient posture is determined by using accelerometer and gyroscope data from inertial control unit placed in autonomous expandable tumor prosthesis. By using the created dataset, 48 features are extracted. Then, for optimization, with feature selection, the number of features is reduced to 10. The selected features are processed using the decision tree, the k-nearest neighborhood and support vector machine algorithms. These algorithms were compared with each other using machine learning performance parameters. Accuracy, recall, precision and F-score values are calculated and compared. Consequently, support vector machine is determined as the most successful technique. Then, the model is tested on the experimental setup developed within the scope of the study, and the posture is determined. It is found that with this system, in the presence of a load on the prosthesis, it can be accurately detected at a rate of 97.1% (the recall parameter). |
References | | | Cited By |
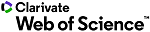
Web of Science® Times Cited: 0
View record in Web of Science® [View]
View Related Records® [View]
Updated today
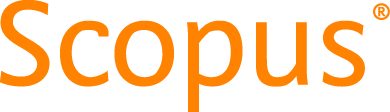
SCOPUS® Times Cited: 2
View record in SCOPUS® [Free preview]
View citations in SCOPUS® [Free preview]
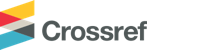
[1] Attitude Detection Classification based on Feature Selection and Integrated Machine Learning Model, Zou, Yonglin, Liu, Xuefen, Liao, Ziqi, Li, Zhi, Scientific Journal of Technology, ISSN 2688-8645, Issue 3, Volume 5, 2023.
Digital Object Identifier: 10.54691/sjt.v5i3.4491 [CrossRef]
[2] Tomato leaf color as predictor of soil moisture value using machine learning techniques, Atanasov, Svetoslav, Harizanova-Petrova, Bilyana, Petrova, Radost, Scientific Horizons, ISSN 2709-8877, Issue 2, Volume 26, 2023.
Digital Object Identifier: 10.48077/scihor.26(2).2023.31-42 [CrossRef]
Disclaimer: All information displayed above was retrieved by using remote connections to respective databases. For the best user experience, we update all data by using background processes, and use caches in order to reduce the load on the servers we retrieve the information from. As we have no control on the availability of the database servers and sometimes the Internet connectivity may be affected, we do not guarantee the information is correct or complete. For the most accurate data, please always consult the database sites directly. Some external links require authentication or an institutional subscription.
Web of Science® is a registered trademark of Clarivate Analytics, Scopus® is a registered trademark of Elsevier B.V., other product names, company names, brand names, trademarks and logos are the property of their respective owners.
Faculty of Electrical Engineering and Computer Science
Stefan cel Mare University of Suceava, Romania
All rights reserved: Advances in Electrical and Computer Engineering is a registered trademark of the Stefan cel Mare University of Suceava. No part of this publication may be reproduced, stored in a retrieval system, photocopied, recorded or archived, without the written permission from the Editor. When authors submit their papers for publication, they agree that the copyright for their article be transferred to the Faculty of Electrical Engineering and Computer Science, Stefan cel Mare University of Suceava, Romania, if and only if the articles are accepted for publication. The copyright covers the exclusive rights to reproduce and distribute the article, including reprints and translations.
Permission for other use: The copyright owner's consent does not extend to copying for general distribution, for promotion, for creating new works, or for resale. Specific written permission must be obtained from the Editor for such copying. Direct linking to files hosted on this website is strictly prohibited.
Disclaimer: Whilst every effort is made by the publishers and editorial board to see that no inaccurate or misleading data, opinions or statements appear in this journal, they wish to make it clear that all information and opinions formulated in the articles, as well as linguistic accuracy, are the sole responsibility of the author.