4/2021 - 6 |
Machine Learning Enhanced Entropy-Based Network Anomaly DetectionTIMCENKO, V.![]() ![]() ![]() ![]() ![]() ![]() |
View the paper record and citations in ![]() |
Click to see author's profile in ![]() ![]() ![]() |
Download PDF ![]() |
Author keywords
clustering algorithms, data flow computing, entropy, intrusion detection, machine learning
References keywords
detection(22), network(21), security(10), intrusion(10), data(10), anomaly(10), systems(9), learning(8), entropy(8), machine(6)
Blue keywords are present in both the references section and the paper title.
About this article
Date of Publication: 2021-11-30
Volume 21, Issue 4, Year 2021, On page(s): 51 - 60
ISSN: 1582-7445, e-ISSN: 1844-7600
Digital Object Identifier: 10.4316/AECE.2021.04006
Web of Science Accession Number: 000725107100006
SCOPUS ID: 85122239638
Abstract
The advanced development of new technologies and heterogeneous environments relies on the proper processing of large data volumes, and accurate and fast response of real-time applications. Such circumstances provide a fertile ground for the appearance of diverse security concerns, thus challenging the scientific community for building more reliable and efficient Network Anomaly Detection Systems. This research proposes a comprehensive flow-based anomaly detection architecture, which encompasses techniques for entropy-based data processing and machine learning-based attack detection. It encompasses several attack categories and relies on the use of modelled and synthetically generated traffic patterns for Port Scan, Network Scan, DDoS amplification, flood, and dictionary attacks. The entropy-based analysis is used for easier detection of the hidden traffic patterns, as it can capture the behaviour of the biggest contributors, and of a large number of minor appearances in the feature distribution. The unusual traffic is then processed by the use of unsupervised machine learning algorithms. The approach is verified with datasets based on real network traffic, synthetically generated attack traffic instances and botnet traffic. The architecture is an original solution, planned for further real-network application, targeting the possible support for a range of different use cases. |
References | | | Cited By |
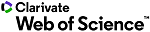
Web of Science® Times Cited: 3 [View]
View record in Web of Science® [View]
View Related Records® [View]
Updated today
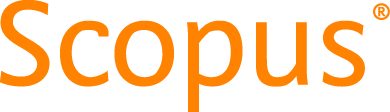
SCOPUS® Times Cited: 5
View record in SCOPUS® [Free preview]
View citations in SCOPUS® [Free preview]
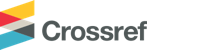
[1] Biometric Identification Advances: Unimodal to Multimodal Fusion of Face, Palm, and Iris Features, KADHIM, O. N., ABDULAMEER, M. H., Advances in Electrical and Computer Engineering, ISSN 1582-7445, Issue 1, Volume 24, 2024.
Digital Object Identifier: 10.4316/AECE.2024.01010 [CrossRef] [Full text]
[2] A Novel Approach to Speech Enhancement Based on Deep Neural Networks, SALEHI, M., MIRZAKUCHAKI, S., Advances in Electrical and Computer Engineering, ISSN 1582-7445, Issue 2, Volume 22, 2022.
Digital Object Identifier: 10.4316/AECE.2022.02009 [CrossRef] [Full text]
[3] Security-ANODR for provisioning of neighbour security in MANETs, Uppe, Nanaji, Mohan, Rao C. P. V. N. J., i-manager’s Journal on Wireless Communication Networks, ISSN 2319-4839, Issue 2, Volume 12, 2024.
Digital Object Identifier: 10.26634/jwcn.12.2.20868 [CrossRef]
[4] A novel method for local anomaly detection of time series based on multi entropy fusion, Wang, Gangjin, Wei, Daijun, Li, Xiangbo, Wang, Ningkui, Physica A: Statistical Mechanics and its Applications, ISSN 0378-4371, Issue , 2023.
Digital Object Identifier: 10.1016/j.physa.2023.128593 [CrossRef]
[5] Yapay zeka tarafından kontrol edilen yeni bir termoelektrik CPU soğutma sistemi, UMUT, İlhan, AKAL, Dinçer, Gazi Üniversitesi Mühendislik Mimarlık Fakültesi Dergisi, ISSN 1300-1884, Issue 1, Volume 39, 2023.
Digital Object Identifier: 10.17341/gazimmfd.1150632 [CrossRef]
[6] Hybrid Machine Learning Traffic Flows Analysis for Network Attacks Detection, Timcenko, Valentina, Gajin, Slavko, 2022 30th Telecommunications Forum (TELFOR), ISBN 978-1-6654-7273-9, 2022.
Digital Object Identifier: 10.1109/TELFOR56187.2022.9983780 [CrossRef]
Disclaimer: All information displayed above was retrieved by using remote connections to respective databases. For the best user experience, we update all data by using background processes, and use caches in order to reduce the load on the servers we retrieve the information from. As we have no control on the availability of the database servers and sometimes the Internet connectivity may be affected, we do not guarantee the information is correct or complete. For the most accurate data, please always consult the database sites directly. Some external links require authentication or an institutional subscription.
Web of Science® is a registered trademark of Clarivate Analytics, Scopus® is a registered trademark of Elsevier B.V., other product names, company names, brand names, trademarks and logos are the property of their respective owners.
Faculty of Electrical Engineering and Computer Science
Stefan cel Mare University of Suceava, Romania
All rights reserved: Advances in Electrical and Computer Engineering is a registered trademark of the Stefan cel Mare University of Suceava. No part of this publication may be reproduced, stored in a retrieval system, photocopied, recorded or archived, without the written permission from the Editor. When authors submit their papers for publication, they agree that the copyright for their article be transferred to the Faculty of Electrical Engineering and Computer Science, Stefan cel Mare University of Suceava, Romania, if and only if the articles are accepted for publication. The copyright covers the exclusive rights to reproduce and distribute the article, including reprints and translations.
Permission for other use: The copyright owner's consent does not extend to copying for general distribution, for promotion, for creating new works, or for resale. Specific written permission must be obtained from the Editor for such copying. Direct linking to files hosted on this website is strictly prohibited.
Disclaimer: Whilst every effort is made by the publishers and editorial board to see that no inaccurate or misleading data, opinions or statements appear in this journal, they wish to make it clear that all information and opinions formulated in the articles, as well as linguistic accuracy, are the sole responsibility of the author.